NeetCode 150 全問の解き方とポイントまとめ Heap/Priority Queue
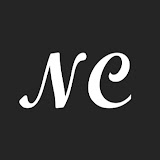
Heap / Priority Queue
本記事は以下記事の一部です。
NeetCode 150 全問の解き方とポイントまとめ & 感想
Heapは比較的シンプルなデータ構造で、Greedyっぽい実装との組み合わせで発展的な問題で使われることが多いので必須。ダイクストラとかトポロジカルソートとかでも使う。
Nの長さの配列をHeap化する時の時間計算量はO(N)で、最小値の参照はO(1)、最小値の取り出しはLog Nとなる。
64. Kth Largest Element In a Stream
Link | https://neetcode.io/problems/kth-largest-integer-in-a-stream |
Difficulty (Easy, Medium, Hard) | Easy |
Time Complexity | 初期化は O(N) 追加はO(Log K) |
Space Complexity | O(K) |
The personal need for review (1 ~ 10) | 3 |
Memo |
|
Problem
Design a class to find the
kth
largest integer in a stream of values, including duplicates. E.g. the2nd
largest from [1, 2, 3, 3] is3
. The stream is not necessarily sorted.Implement the following methods:
constructor(int k, int[] nums)
Initializes the object given an integerk
and the stream of integersnums
.int add(int val)
Adds the integerval
to the stream and returns thekth
largest integer in the stream.Example 1:
Input: ["KthLargest", [3, [1, 2, 3, 3]], "add", [3], "add", [5], "add", [6], "add", [7], "add", [8]] Output: [null, 3, 3, 3, 5, 6] Explanation: KthLargest kthLargest = new KthLargest(3, [1, 2, 3, 3]); kthLargest.add(3); // return 3 kthLargest.add(5); // return 3 kthLargest.add(6); // return 3 kthLargest.add(7); // return 5 kthLargest.add(8); // return 6
Copy
Constraints:
1 <= k <= 1000
0 <= nums.length <= 1000
-1000 <= nums[i] <= 1000
-1000 <= val <= 1000
- There will always be at least
k
integers in the stream when you search for thekth
integer.
Solution
import heapq
class KthLargest:
def __init__(self, k: int, nums: List[int]):
self.k = k
self.min_heap = nums
heapq.heapify(self.min_heap)
# Kよりも長い場合はKの長さになるまで最小値をpopする
# こうすることで、self.min_heap[0]がK番目に大きい数字になる
while len(self.min_heap) > self.k:
heapq.heappop(self.min_heap)
def add(self, val: int) -> int:
heapq.heappush(self.min_heap, val)
if len(self.min_heap) > self.k:
heapq.heappop(self.min_heap)
return self.min_heap[0]
65. Last Stone Weight
Link | |
Difficulty (Easy, Medium, Hard) | Easy |
Time Complexity | O (N Log N) |
Space Complexity | O(N) |
The personal need for review (1 ~ 10) | 3 |
Memo |
|
Problem
Design a class to find the
kth
largest integer in a stream of values, including duplicates. E.g. the2nd
largest from [1, 2, 3, 3] is3
. The stream is not necessarily sorted.Implement the following methods:
constructor(int k, int[] nums)
Initializes the object given an integerk
and the stream of integersnums
.int add(int val)
Adds the integerval
to the stream and returns thekth
largest integer in the stream.Example 1:
Input: ["KthLargest", [3, [1, 2, 3, 3]], "add", [3], "add", [5], "add", [6], "add", [7], "add", [8]] Output: [null, 3, 3, 3, 5, 6] Explanation: KthLargest kthLargest = new KthLargest(3, [1, 2, 3, 3]); kthLargest.add(3); // return 3 kthLargest.add(5); // return 3 kthLargest.add(6); // return 3 kthLargest.add(7); // return 5 kthLargest.add(8); // return 6
Copy
Constraints:
1 <= k <= 1000
0 <= nums.length <= 1000
-1000 <= nums[i] <= 1000
-1000 <= val <= 1000
- There will always be at least
k
integers in the stream when you search for thekth
integer.
Solution
import heapq
class Solution:
def lastStoneWeight(self, stones: List[int]) -> int:
min_heap = [-s for s in stones]
heapq.heapify(min_heap)
while len(min_heap) > 1:
largest = heapq.heappop(min_heap)
second = heapq.heappop(min_heap)
diff = abs(largest - second)
if diff > 0:
heapq.heappush(min_heap, -diff)
if min_heap:
return -min_heap[0]
else:
return 0
66. K Closest Points to Origin
Link | |
Difficulty (Easy, Medium, Hard) | Medium |
Time Complexity | O (K Log N) |
Space Complexity | O(N) |
The personal need for review (1 ~ 10) | 3 |
Memo |
|
Problem
You are given an 2-D array
points
wherepoints[i] = [xi, yi]
represents the coordinates of a point on an X-Y axis plane. You are also given an integerk
.Return the
k
closest points to the origin(0, 0)
.The distance between two points is defined as the Euclidean distance (
sqrt((x1 - x2)^2 + (y1 - y2)^2))
.You may return the answer in any order.
Example 1:
Input: points = [[0,2],[2,2]], k = 1 Output: [[0,2]]
Copy
Explanation : The distance between
(0, 2)
and the origin(0, 0)
is2
. The distance between(2, 2)
and the origin issqrt(2^2 + 2^2) = 2.82842
. So the closest point to the origin is(0, 2)
.Example 2:
Input: points = [[0,2],[2,0],[2,2]], k = 2 Output: [[0,2],[2,0]]
Copy
Explanation: The output
[2,0],[0,2]
would also be accepted.Constraints:
1 <= k <= points.length <= 1000
-100 <= points[i][0], points[i][1] <= 100
Solution
class Solution:
def calcEuclideanDistance(self, x1: int, y1: int, x2: int, y2: int) -> int:
return math.sqrt((x1-x2)**2 + (y1-y2)**2)
def kClosest(self, points: List[List[int]], k: int) -> List[List[int]]:
res = []
min_heap = [] # (distance, x, y)
for x2, y2 in points:
x1, y1 = 0, 0
distance = self.calcEuclideanDistance(x1, y1, x2, y2)
min_heap.append((distance, x2, y2))
heapq.heapify(min_heap)
while k > 0:
distance, x, y = heapq.heappop(min_heap)
res.append([x, y])
k-=1
return res
67. Kth Largest Element In An Array
Link | https://neetcode.io/problems/kth-largest-element-in-an-array |
Difficulty (Easy, Medium, Hard) | Medium |
Time Complexity | |
Space Complexity | |
The personal need for review (1 ~ 10) | 5 |
Memo |
|
Problem
Given an unsorted array of integers
nums
and an integerk
, return thekth
largest element in the array.By
kth
largest element, we mean thekth
largest element in the sorted order, not thekth
distinct element.Follow-up: Can you solve it without sorting?
Example 1:
Input: nums = [2,3,1,5,4], k = 2 Output: 4
Copy
Example 2:
Input: nums = [2,3,1,1,5,5,4], k = 3 Output: 4
Copy
Constraints:
1 <= k <= nums.length <= 10000
-1000 <= nums[i] <= 1000
Solution
import random
class Solution:
def findKthLargest(self, nums: List[int], k: int) -> int:
m = random.choice(nums)
smaller = [n for n in nums if n < m]
same = [n for n in nums if n == m]
larger = [n for n in nums if n > m]
if k <= len(larger):
return self.findKthLargest(larger, k)
elif k > len(larger) + len(same):
return self.findKthLargest(smaller, k - len(larger) - len(same))
else:
return same[0]
68. Task Scheduler
Link | |
Difficulty (Easy, Medium, Hard) | Medium |
Time Complexity | O(M Log K) M: タスクのカウント K: 異なるタスクの数 |
Space Complexity | O (K) |
The personal need for review (1 ~ 10) | 6 |
Memo |
|
Problem
Given an unsorted array of integers
nums
and an integerk
, return thekth
largest element in the array.By
kth
largest element, we mean thekth
largest element in the sorted order, not thekth
distinct element.Follow-up: Can you solve it without sorting?
Example 1:
Input: nums = [2,3,1,5,4], k = 2 Output: 4
Copy
Example 2:
Input: nums = [2,3,1,1,5,5,4], k = 3 Output: 4
Copy
Constraints:
1 <= k <= nums.length <= 10000
-1000 <= nums[i] <= 1000
Solution
import random
class Solution:
def findKthLargest(self, nums: List[int], k: int) -> int:
m = random.choice(nums)
smaller = [n for n in nums if n < m]
same = [n for n in nums if n == m]
larger = [n for n in nums if n > m]
if k <= len(larger):
return self.findKthLargest(larger, k)
elif k > len(larger) + len(same):
return self.findKthLargest(smaller, k - len(larger) - len(same))
else:
return same[0]
69. Design Twitter
Link | |
Difficulty (Easy, Medium, Hard) | Medium |
Time Complexity | O(F T) + O(N) + O(K Log N) |
Space Complexity | O(U^2) + O(U T) |
The personal need for review (1 ~ 10) | 6 |
Memo |
|
Problem
Given an unsorted array of integers
nums
and an integerk
, return thekth
largest element in the array.By
kth
largest element, we mean thekth
largest element in the sorted order, not thekth
distinct element.Follow-up: Can you solve it without sorting?
Example 1:
Input: nums = [2,3,1,5,4], k = 2 Output: 4
Copy
Example 2:
Input: nums = [2,3,1,1,5,5,4], k = 3 Output: 4
Copy
Constraints:
1 <= k <= nums.length <= 10000
-1000 <= nums[i] <= 1000
Solution
from collections import defaultdict, deque
import heapq
class Twitter:
def __init__(self):
# two hashmap
# follower, followee
# user, tweet
# time
self.follow_map = defaultdict(set)
self.tweet_map = defaultdict(list) # (time, tweetId)
self.time = 0
def postTweet(self, userId: int, tweetId: int) -> None:
self.tweet_map[userId].append((self.time, tweetId))
self.time += 1
def getNewsFeed(self, userId: int) -> List[int]:
self.follow_map[userId].add(userId)
all_tweets = []
for followee in self.follow_map[userId]:
for time, tweetId in self.tweet_map[followee]:
all_tweets.append((-time, tweetId))
heapq.heapify(all_tweets)
k = 10
queue = deque()
res = []
while all_tweets and k > 0:
time, tweetId = heapq.heappop(all_tweets)
res.append(tweetId)
queue.append((time, tweetId))
k-=1
for time, tweetId in queue:
heapq.heappush(all_tweets, (time, tweetId))
return res
def follow(self, followerId: int, followeeId: int) -> None:
if followeeId not in self.follow_map[followerId]:
self.follow_map[followerId].add(followeeId)
def unfollow(self, followerId: int, followeeId: int) -> None:
if followeeId in self.follow_map[followerId]:
self.follow_map[followerId].remove(followeeId)
70. Find Median From Data Stream
Link | |
Difficulty (Easy, Medium, Hard) | Hard |
Time Complexity | O(Log N) |
Space Complexity | O(N) |
The personal need for review (1 ~ 10) | 8 |
Memo |
|
Problem
The median is the middle value in a sorted list of integers. For lists of even length, there is no middle value, so the median is the mean of the two middle values.
For example:
- For
arr = [1,2,3]
, the median is2
.- For
arr = [1,2]
, the median is(1 + 2) / 2 = 1.5
Implement the MedianFinder class:
MedianFinder()
initializes theMedianFinder
object.void addNum(int num)
adds the integernum
from the data stream to the data structure.double findMedian()
returns the median of all elements so far.Example 1:
Input: ["MedianFinder", "addNum", "1", "findMedian", "addNum", "3" "findMedian", "addNum", "2", "findMedian"] Output: [null, null, 1.0, null, 2.0, null, 2.0] Explanation: MedianFinder medianFinder = new MedianFinder(); medianFinder.addNum(1); // arr = [1] medianFinder.findMedian(); // return 1.0 medianFinder.addNum(3); // arr = [1, 3] medianFinder.findMedian(); // return 2.0 medianFinder.addNum(2); // arr[1, 2, 3] medianFinder.findMedian(); // return 2.0
Copy
Constraints:
-100,000 <= num <= 100,000
findMedian
will only be called after adding at least one integer to the data structure.
Solution
import heapq
class MedianFinder:
def __init__(self):
# store smaller half
self.max_heap = []
# store larger half
self.min_heap = []
def addNum(self, num: int) -> None:
if self.max_heap and -self.max_heap[0] >= num:
heapq.heappush(self.max_heap, -num)
else:
heapq.heappush(self.min_heap, num)
if len(self.max_heap) > len(self.min_heap) + 1:
smallest_max_h = heapq.heappop(self.max_heap)
heapq.heappush(self.min_heap, -smallest_max_h)
if len(self.min_heap) > len(self.max_heap) + 1:
largest_min_h = heapq.heappop(self.min_heap)
heapq.heappush(self.max_heap, -largest_min_h)
def findMedian(self) -> float:
if len(self.min_heap) > len(self.max_heap):
return self.min_heap[0]
if len(self.max_heap) > len(self.min_heap):
return -self.max_heap[0]
return (self.min_heap[0] + -self.max_heap[0]) / 2